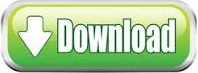

#Tessellation definition arcgis software
Loose programming is an emerging software engineering paradigm that focuses on automatically synthesizing programs based on loose specifications (Lamprecht, Naujokat, Margaria, & Steffen, 2010). Unfortunately, this has hardly been done in previous works (see Section 2). That means the actual synthesis process of GIS workflows itself needs to be taken into focus. For example, in the task above, a different yet equally valid approach might involve analyzing land use patches instead of trees. Yet, generating a workflow involves creativity in synthesizing an answer, which goes beyond information retrieval. Finally, geo‐operator thesauri and pairwise service matching (Brauner, 2015) may help retrieve tools for a given purpose. For example, while the different spatial point interpolation operations, such as inverse distance weighting (IDW) and Kriging, appear as separate functions in ArcGIS ( ‐app/tool‐reference/3d‐analyst/an‐overview‐of‐the‐raster‐interpolation‐toolset.htm), they are merely parameters of a single function in R ( ‐interpolation.html). Furthermore, since different tool sets capture similar functionality at different levels of granularity, it seems hard to design a general tool ontology (see Albrecht, 1998). For instance, in our example above, the point vector data remains ambiguous without human interpretation as to whether it can be used for density estimation or rather for spatial interpolation. The state‐of‐the‐art data types, such as vector and raster, do not capture important analytic constraints. For one, it remained unclear at what level GIS data should best be described (Hofer, Mäs, et al., 2017). However, this approach had limited success for several reasons. In the past, researchers have suggested semantics‐based geoservice descriptions and service matchings to help analysts achieve a computational goal (see Section 2 see also Hofer, Mäs, Brauner, & Bernard, 2017). Producing such workflows is difficult and hard to automate, given the level of expertise and the growing amount of tools and data sources to be familiar with (Scheider, Nyamsuren, Kruiger, and Xu, 2020).Įxample of a geo‐analytical workflow in GISĪn essential scientific challenge for geographic information science is therefore to understand what kind of knowledge is needed to automate this task.
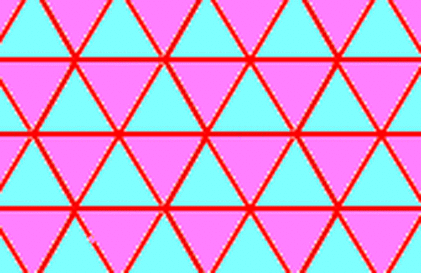
Different kinds of GIS tools (e.g., kernel density, distance, and spatial interpolation) might be applied to different kinds of input data (e.g., an inventory of trees as points, maps of land use, and pointwise measures of air quality) to design a valid workflow for assessing the exposure of the run. For example, in the context of health geography, suppose our task is to assess how much a person (say, Tom) is exposed to health‐relevant environmental factors when running through the city of Amsterdam (Figure (Figure1). This includes background knowledge of data sources, about their semantics, formats and data qualities, knowledge of GIS functions, as well as a sound understanding of the analytic goal to be pursued. Results show that CCD concepts significantly improve the precision of workflow synthesis.Ĭreating useful workflows for analysis with geographic information systems (GIS) requires a lot of expertise. We measure the quality of automatically synthesized workflows against a benchmark generated from common data types.

In this article we synthesize workflows by annotating GIS tools with these types, specifying a range of common analytical tasks taken from an urban livability scenario. However, to date, it is unknown what gain in precision and workflow quality can be expected. The core concept data types (CCD) ontology is a semantic type system that can be used to constrain GIS functions for workflow synthesis. Recently, core concepts of spatial information and related geo‐analytical concepts were proposed as a way to add the required abstraction level to current geodata models. To synthesize workflows of high quality for a geo‐analytical task, the semantic type system needs to reflect knowledge of geographic information systems (GIS) at a level that is deep enough to capture geo‐analytical concepts and intentions, yet shallow enough to generalize over GIS implementations. Data transformations are left underspecified, leaving out procedural details and exploiting knowledge about the applicability of functions to data types. Loose programming enables analysts to program with concepts instead of procedural code.
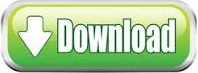